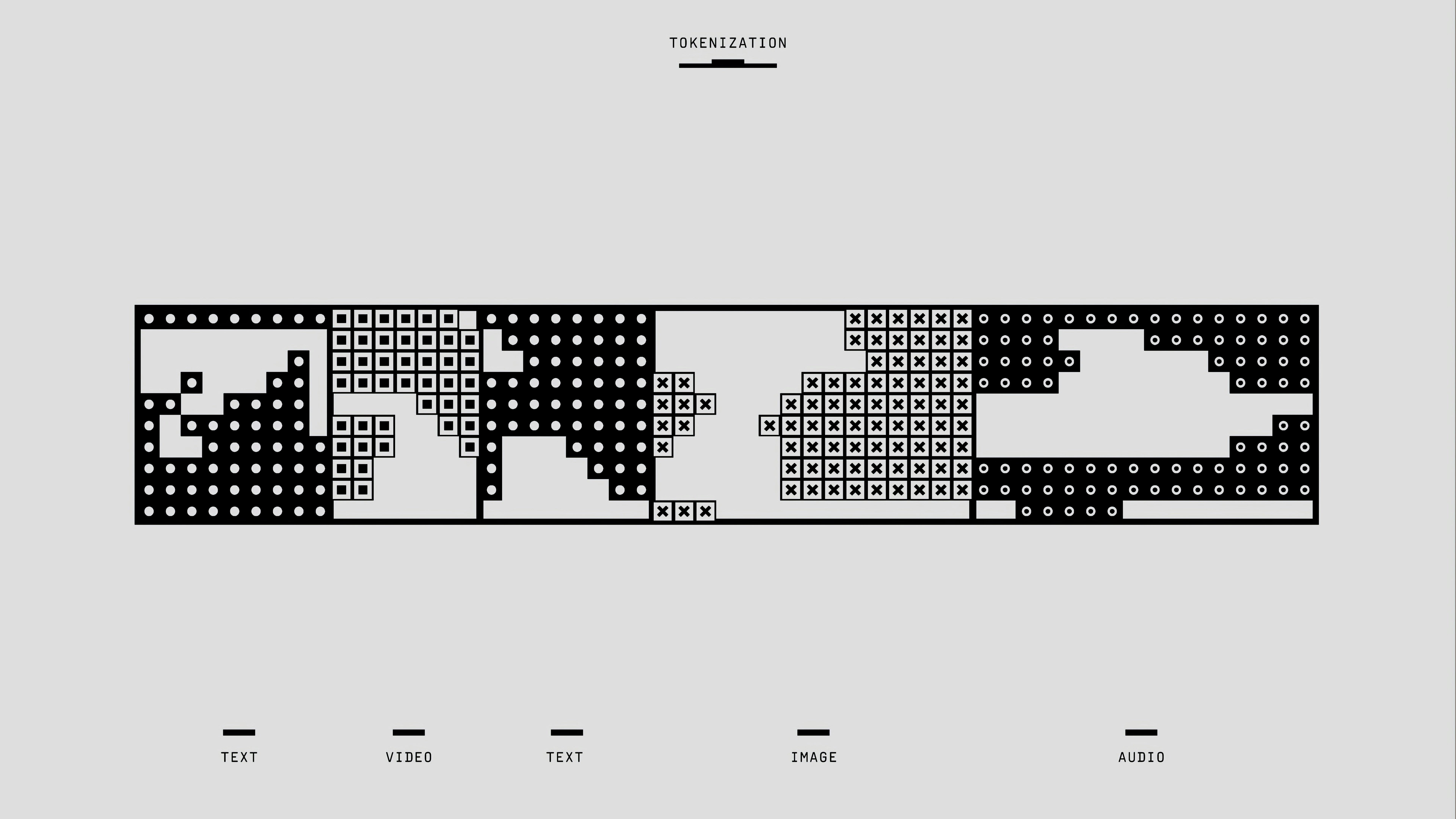
Quantum-Enhanced AI in Semiconductors—Powering the Next Era of Microelectronics
The semiconductor industry is the backbone of modern technology, underpinning everything from smartphones and cloud servers to automotive electronics and advanced robotics. Over decades, semiconductor manufacturing has followed Moore’s Law, consistently increasing transistor density while reducing cost. Yet as we approach sub-nanometre scales, conventional semiconductor techniques face monumental challenges in design, fabrication, and materials science. Coupled with soaring demand for more powerful and energy-efficient chips, the industry must explore breakthroughs beyond classical approaches.
Enter quantum computing—a frontier technology harnessing the strange properties of quantum mechanics to perform certain computations at potentially exponential speed-ups. Paired with Artificial Intelligence (AI), quantum hardware might expedite complex tasks like design-space exploration, lithography simulation, advanced testing, and yield optimisation. If harnessed effectively, quantum-enhanced AI could help transcend the current limits of chip performance and open new architectural paradigms for next-gen electronics.
In this article, we will:
Examine the current state of the semiconductor industry, including why classical methods are hitting bottlenecks.
Introduce quantum computing fundamentals—why qubits differ from bits and how they could boost AI.
Explore how quantum-enhanced AI can address semiconductor challenges, from chip design to supply chain optimisation.
Highlight real-world pilot projects, potential near-term successes, and the main hurdles (like noisy quantum hardware).
Discuss the emerging career paths and skill sets that will be crucial for professionals aiming to stand out in an era of quantum-driven semiconductor innovation.
Whether you’re a semiconductor engineer, a data scientist in chip R&D, or simply curious about the intersection of hardware and quantum technologies, read on. Quantum-enhanced AI might reshape every aspect of semiconductor design, fabrication, and testing, propelling electronics into uncharted territory.
The semiconductor industry is the backbone of modern technology, underpinning everything from smartphones and cloud servers to automotive electronics and advanced robotics. Over decades, semiconductor manufacturing has followed Moore’s Law, consistently increasing transistor density while reducing cost. Yet as we approach sub-nanometre scales, conventional semiconductor techniques face monumental challenges in design, fabrication, and materials science. Coupled with soaring demand for more powerful and energy-efficient chips, the industry must explore breakthroughs beyond classical approaches.
Enter quantum computing—a frontier technology harnessing the strange properties of quantum mechanics to perform certain computations at potentially exponential speed-ups. Paired with Artificial Intelligence (AI), quantum hardware might expedite complex tasks like design-space exploration, lithography simulation, advanced testing, and yield optimisation. If harnessed effectively, quantum-enhanced AI could help transcend the current limits of chip performance and open new architectural paradigms for next-gen electronics.
In this article, we will:
Examine the current state of the semiconductor industry, including why classical methods are hitting bottlenecks.
Introduce quantum computing fundamentals—why qubits differ from bits and how they could boost AI.
Explore how quantum-enhanced AI can address semiconductor challenges, from chip design to supply chain optimisation.
Highlight real-world pilot projects, potential near-term successes, and the main hurdles (like noisy quantum hardware).
Discuss the emerging career paths and skill sets that will be crucial for professionals aiming to stand out in an era of quantum-driven semiconductor innovation.
Whether you’re a semiconductor engineer, a data scientist in chip R&D, or simply curious about the intersection of hardware and quantum technologies, read on. Quantum-enhanced AI might reshape every aspect of semiconductor design, fabrication, and testing, propelling electronics into uncharted territory.
1. The Semiconductor Landscape: Achievements and Pressures
1.1 A Legacy of Continuous Improvement
From the invention of the transistor in the 1940s through to today’s multi-billion-transistor microprocessors, the semiconductor sector has fuelled the digital age. Key enablers include:
Shrinking Process Nodes: 14nm → 7nm → 5nm → 3nm and beyond, driving faster, smaller, more energy-efficient chips.
EUV Lithography: Advanced lithography equipment enabling precise patterning at near-atomic scales.
Complex Chip Architectures: Multi-core CPUs, GPUs, FPGAs, and AI accelerators designed to handle different workloads efficiently.
Manufacturing Scalability: Foundries producing millions of chips monthly, supporting global electronics markets.
Yet as transistors approach quantum-mechanical size limits, conventional methods face diminishing returns, rising costs, and reliability issues.
1.2 Challenges Confronting the Industry
Design Complexity: Modern system-on-chips (SoCs) integrate billions of components, making manual design verification and layout an enormous undertaking.
Manufacturing Variability: As process nodes shrink, even minuscule variations in temperature, impurities, or lithographic alignment can degrade yields.
Materials Innovation Needs: Traditional silicon-based semiconductors must be supplemented with novel materials (e.g., GaN, SiC, 2D materials) to continue performance gains.
Emerging AI Demands: AI and data analytics drive intense computational requirements, demanding new architectures (in-memory computing, neuromorphic chips) that deviate from standard designs.
Quantum computing—particularly when integrated with AI—may offer fresh solutions, accelerating design cycles, refining manufacturing processes, and enabling new materials discovery vital to the semiconductor future.
2. Quantum Computing Basics
2.1 Qubits vs. Classical Bits
Classical bits can be 0 or 1. Quantum computing uses qubits, which exploit quantum effects:
Superposition: A qubit can represent 0 and 1 simultaneously, opening parallel computational pathways.
Entanglement: Two or more qubits can become correlated such that measuring one instantly influences the other, enabling complex operations far beyond classical logic gates.
These attributes allow quantum machines to tackle specific classes of problems—like huge optimisation tasks or simulating quantum states—much faster than classical computers.
2.2 The NISQ Era
Today’s machines are in the NISQ (Noisy Intermediate-Scale Quantum) phase, featuring:
Limited Qubit Counts: Tens or hundreds of qubits, often with short coherence times.
Noise Sensitivity: Qubit states degrade easily, introducing computational errors.
Cloud-Based Access: Major providers (IBM, Amazon, Microsoft, Google) offer quantum hardware via cloud services, as on-premises systems remain rare and expensive.
While we’ve yet to see broad quantum advantage for everyday tasks, dedicated use cases in chemistry, materials science, and large-scale optimisation show promise—relevant to many steps in semiconductor R&D.
3. Quantum-Enhanced AI: Bridging Two Frontiers
3.1 Why Merge Quantum and AI for Semiconductors?
Quantum-enhanced AI refers to the synergy of quantum computing with machine learning algorithms, potentially delivering:
Faster Computations: Specific subroutines (like matrix inversion, large-scale optimisation, or sampling) can see major speed-ups on quantum hardware.
New ML Architectures: Quantum neural networks might compress or explore design spaces more effectively, critical for chip layout or materials exploration.
Efficient Searching: Large combinatorial problems—like transistor placement, routing, or manufacturing scheduling—benefit from quantum parallelism.
3.2 Hybrid Classical-Quantum Workflows
Given the current NISQ constraints, real-world solutions adopt hybrid approaches: classical systems do the bulk of data handling, orchestrating, and simpler ML tasks, while quantum computers execute the most complex computational kernels. This arrangement mirrors how HPC clusters leverage GPUs or FPGAs for specialised tasks in classical computing pipelines.
3.3 Potential Impact on Semiconductor Innovations
From advanced lithography simulations to solving intricate yield-optimisation problems, quantum-enhanced AI might significantly reduce R&D cycles, cut manufacturing costs, and let engineers push beyond what classical HPC can accomplish—revitalising Moore’s Law or guiding its evolution into new paradigms.
4. Use Cases: Quantum-Enhanced AI in the Semiconductor Sector
4.1 Chip Design & EDA (Electronic Design Automation)
Designing modern chips involves Electronic Design Automation (EDA) tools for tasks like schematic capture, place-and-route, and timing verification:
Placement & Routing: Positioning billions of components on silicon while ensuring minimal signal delay is a combinatorial puzzle. Quantum-assisted optimisers (like QAOA) may drastically reduce compute times.
Timing Closure: Balancing clock domains, latencies, and power constraints is another complex process that might be tackled faster with quantum subroutines.
DFT (Design for Test): AI-based solutions for test pattern generation and fault coverage can offload large search spaces to quantum hardware.
4.2 Advanced Materials & Process Nodes
Materials science is integral to pushing beyond conventional silicon:
Quantum Chemistry Simulations: Evaluate new materials’ band structures or doping behaviours with greater accuracy—key for gallium nitride (GaN), silicon carbide (SiC), or emerging 2D semiconductors like graphene.
Process Optimisation: Predicting how materials react under lithographic or etch processes can be done with quantum-enhanced ML, reducing trial-and-error and R&D budgets.
Thermal Management Solutions: Explore new alloys or chip packaging techniques with quantum-level simulations of heat conduction at nano scales.
4.3 Manufacturing and Yield Optimisation
Semiconductor fabrication (fabs) are multi-billion-pound facilities with intricate multi-step processes:
Process Control & Scheduling: Quantum-based optimisation can schedule thousands of manufacturing steps across lines, minimising downtime and resource conflicts.
Defect Detection & Classification: AI-driven inspection systems rely on high-resolution image analysis. Quantum subroutines could speed pattern recognition or anomaly detection for wafer-level defects.
Yield Learning: Rapidly correlating equipment logs, wafer data, and end-of-line test results can be formidable. Quantum ML might highlight patterns missed by classical analytics, boosting yields in real-time.
4.4 Supply Chain and Logistics
The semiconductor supply chain is global and fragile—recent chip shortages highlight how crucial efficient logistics can be:
Inventory Forecasting: Quantum-enhanced ML can handle multi-factor demand signals across consumer electronics, automotive, and data centre markets.
Warehouse & Distribution Optimisation: Minimising shipping times, storage constraints, and distribution routes can significantly reduce lead times.
Risk Assessment: Identifying supplier vulnerabilities or anticipating production line disruptions might be more efficient with quantum-accelerated scenario modelling.
4.5 Next-Gen Chip Architectures (Quantum Processors)
Ironically, quantum computing itself depends on advanced semiconductor processes:
Quantum Dot Manufacturing: AI guiding the precise doping and structuring of quantum dots for hardware qubits.
Cryogenic CMOS: Designing control electronics that function at near-zero temperatures is a cutting-edge challenge, potentially tackled by quantum-based design exploration.
3D/Advanced Packaging: Combining classical and quantum components in 3D stacked architectures for integrated quantum systems.
5. Challenges to Overcome
5.1 Noisy Hardware and Limited Qubit Counts
Current quantum computers struggle with:
Error Rates: Qubits quickly lose coherence, introducing computational errors.
Scalability: Tasks requiring large qubit arrays exceed current hardware capabilities.
Unpredictable Latencies: Running quantum computations in the cloud can hamper real-time or iterative processes in EDA or manufacturing control loops.
5.2 Data Handling and Encoding
Modern chip design and manufacturing produce enormous datasets:
Efficient Encoding: Translating high-dimensional design or process data into qubit states is non-trivial, often overshadowing theoretical quantum gains.
Hybrid Orchestration: Complex pipelines must coordinate classical HPC and quantum calls, requiring robust infrastructure and APIs.
5.3 Corporate Buy-In and ROI
Semiconductor R&D is expensive and risk-averse:
Proving Quantum Advantage: Pilots must demonstrate real cost savings or speed-ups over classical HPC to justify quantum’s higher costs.
Skill Gaps: Hiring or upskilling engineers and data scientists in quantum computing, AI, and semiconductors poses a major hurdle.
Long Lead Times: From concept to volume production, chip innovation cycles can span years, making immediate quantum ROI uncertain.
5.4 Intellectual Property and Security
Semiconductor IP is extremely valuable. Outsourcing quantum tasks to cloud services might raise concerns about:
Data Confidentiality: Sensitive design or yield data must remain secure from competitor espionage or potential post-quantum cryptography threats.
Patent Complexities: Novel quantum-driven methodologies might require new IP frameworks, complicating licensing and collaboration.
6. Constructing Quantum-Enabled Semiconductor Pipelines
6.1 Typical Hybrid Workflow
Data Preprocessing: EDA, fab data, or design specs are ingested by classical HPC systems.
Quantum Task Offload: Select tasks (e.g., advanced placement optimisation, defect pattern analysis) are delegated to quantum back-ends via cloud APIs (IBM Quantum, Microsoft Azure, Amazon Braket).
Classical-Quantum Integration: Output from the quantum module (e.g., candidate design layouts, yield predictions) is fed into the main pipeline for refinement or further simulation.
Continuous Iteration: As design or process changes arise, the cycle repeats, gradually converging on an optimal chip layout or stable manufacturing process.
6.2 Key Tools & Frameworks
Quantum SDKs: Qiskit (IBM), Cirq (Google), PennyLane (Xanadu) for circuit creation, often integrated into data science workflows.
Traditional EDA Suites: Cadence, Synopsys, Mentor Graphics (Siemens EDA) might eventually add quantum-enabled features or APIs.
ML Platforms: TensorFlow, PyTorch for deep learning, sometimes extended with quantum layers (like TensorFlow Quantum).
Cloud Orchestration: HPC or container-based approaches to manage data flow between classical HPC clusters and quantum resources.
6.3 Best Practices
Pilot Projects: Start with smaller, well-defined sub-problems—like a single block placement or a single wafer’s defect detection—before scaling.
Performance Benchmarking: Regularly compare quantum vs. classical performance to confirm speed-ups or accuracy gains.
Data Minimisation: Only load data subsets that genuinely benefit from quantum acceleration, given overhead in transferring large volumes to quantum hardware.
7. Emerging Roles & Career Prospects
7.1 Quantum EDA Researcher
Algorithm Development: Invent or adapt quantum algorithms for place-and-route, layout verification, or timing closure.
EDA Tool Integration: Collaborate with vendor tools (Cadence, Synopsys) to embed quantum subroutines.
Benchmarking & Validation: Evaluate quantum-driven improvements and ensure results align with physical constraints.
7.2 AI Developer with Quantum Focus (Semiconductors)
Machine Learning Pipelines: Build end-to-end solutions for wafer defect detection, yield analysis, or supply chain optimisation.
Quantum API Management: Write bridging code that calls quantum circuits for large-scale matrix operations or combinatorial tasks.
DevOps & MLOps for Quantum: Oversee version control, testing, and deployment in HPC and cloud environments with quantum endpoints.
7.3 Quantum Process Engineer
Material Simulation Expertise: Use quantum simulations to evaluate doping profiles, new semiconductors, or wafer bonding processes.
Inline & Metrology Data Integration: Link manufacturing logs with quantum-based analytics to predict and prevent yield losses.
Collaboration with Fab Teams: Translate quantum-driven insights into real process changes on production lines.
7.4 Post-Quantum Security Specialist
IP Protection: Ensure safe data transfers to quantum cloud providers, using post-quantum cryptographic protocols.
Supply Chain Encryption: Protect shipments and communications across global supply chains from quantum-based hacking threats.
Regulatory Compliance: Advise on compliance for sensitive defence or telecom chips reliant on advanced security.
8. Challenges and Ethical Dimensions
8.1 Energy Consumption
Quantum computers can be energy-intensive due to cryogenic cooling. Balancing the benefit of potential speed-ups vs. the added power overhead remains important, especially as the semiconductor sector grapples with sustainability.
8.2 IP Ownership & Collaboration
Quantum-led solutions might require collaborations between foundries, EDA vendors, and quantum service providers, raising questions about who owns the resulting IP or how to share data safely.
8.3 Talent Scarcity & Training
Few professionals span quantum computing, AI, and semiconductor knowledge. Companies must invest in training, partnerships, or targeted recruitment. Universities may offer new cross-disciplinary programmes.
8.4 Reliability & Accountability
In critical semiconductor R&D—e.g., chips for aerospace, medical devices—robust verification is vital. Ensuring quantum-driven recommendations align with real physics and constraints is essential. Mistakes can cost billions if a flaw reaches mass production.
9. Future Outlook: 1, 5, and 10 Years
9.1 Near-Term (1–2 Years)
Pilot Prototypes: Universities, consortia, and leading semiconductor firms run small-scale quantum-accelerated tasks (e.g., place-and-route in restricted designs).
Quantum-Enhanced EDA Tools: Early releases from start-ups or tech giants integrating quantum subroutines into classical EDA frameworks.
Skill Development: Growing interest in quantum-semiconductor synergy leads to new training modules and hackathons.
9.2 Mid-Term (3–5 Years)
Incremental Adoption: Demonstrations of genuine time or cost savings in certain design or manufacturing phases encourage broader pilot expansions.
Hardware with a Few Thousand Qubits: Better error correction might enable moderate-scale chip simulations or advanced yield analyses.
Industry Standards Emerge: Collaborative bodies define best practices for quantum-based design, IP protection, and data integration.
9.3 Long-Term (5–10+ Years)
Mainstream Quantum-EDA Integration: Quantum-accelerated AI becomes routine in front-end design, backend layout, and process control.
Radical Materials & Device Concepts: New transistor architectures or post-CMOS paradigms discovered via quantum simulation enter commercial fabrication.
Global Semiconductor Shifts: Nations and corporations leveraging quantum technology gain competitive advantages in HPC, AI, and advanced chip production—reshaping the global electronics supply chain.
10. Conclusion
As we near the physical limits of classical semiconductor fabrication, the future of microelectronics hinges on inventive leaps across design, manufacturing, and materials science. Quantum computing, though still young and imperfect, offers a tantalising path to break classical barriers—particularly when fused with AI in a quantum-enhanced synergy. From accelerating EDA tasks to pinpointing yield bottlenecks in fabs, this technology could revitalise Moore’s Law or even supplant it with novel architectures.
For professionals in semiconductor engineering—whether you focus on design, manufacturing, or supply chain analytics—now is the time to explore quantum basics, engage with pilot studies, and build cross-disciplinary collaborations. The next wave of microelectronics may well be powered by quantum hardware and algorithms, forging breakthroughs that define the digital world for decades.
Ready to advance your career in semiconductors? Seeking roles that combine deep hardware knowledge with emerging quantum and AI skill sets? Visit www.semiconductorjobs.co.uk for the latest opportunities in this rapidly evolving field. By embracing quantum-enhanced AI, you can be part of the vanguard shaping tomorrow’s chips, devices, and computing paradigms—and, in the process, fuel the continued rise of digital innovation worldwide.